Diabetes continues to rise throughout the United States and worldwide. As of 2019, around 463 million people were estimated to have diabetes mellitus, which is 9.3% of the global population.1 Furthermore, estimates show that the prevalence of diabetes mellitus will rise to 578 million people (10.2%) by the year 2030 and 700 million people (10.9%) by the year 2045.1 This increase in the prevalence of diabetes can also lead to a corresponding increase in diabetic complications, including nephropathy, cardiovascular disease, neuropathy, and retinopathy.
Diabetic retinopathy remains a common complication of diabetes mellitus and is one of the leading causes of preventable vision loss both in the United States and globally.2 There are multiple stages of diabetic retinopathy, including mild nonproliferative, moderate nonproliferative, severe nonproliferative, and proliferative diabetic retinopathy. Proliferative diabetic retinopathy signifies the development of neovascularization, and poses high risk of vision threatening complications, including tractional retinal detachment, vitreous hemorrhage, and macular ischemia. Patients in any stage of diabetic retinopathy can also have diabetic macular edema, which in itself can limit vision. Treatment to date consists of good systemic sugar control along with intravitreal anti-VEGF injections, intravitreal steroid injections, focal laser, and/or panretinal photocoagulation.
It is estimated that vision-threatening diabetic retinopathy (vtDR) is present in around 22% of patients with diabetes, with clinically significant macular edema present in about 4% of patients,2 although the numbers can widely vary based on the population and access to care. Regardless of the exact numbers, there is no doubt that diabetic retinopathy remains a significant health burden and cause of visual loss. Early detection of diabetic retinopathy is key, because early treatment and lifestyle modifications can help prevent or minimize vision-threatening complications.
IMPORTANCE OF SCREENING
Diabetes tends to affect young and middle-aged patients, and therefore retinopathy disproportionately affects working-aged adults. There have been numerous studies on noncompliance among diabetic patients.3-6 The reasons for noncompliance are multifactorial, but can be influenced by difficulty in coming to the physician’s office, poor understanding of disease, high treatment burden, and lack of access to care. Screening all diabetic patients for retinopathy is important, because diabetic retinopathy can be asymptomatic or mildly symptomatic even in severe stages of disease. Furthermore, early treatment can lead to significantly improved outcomes throughout the course of the disease.7
ARTIFICIAL INTELLIGENCE FOR THE SCREENING OF DIABETIC RETINOPATHY
There are many programs across the globe to help facilitate diabetic screening, including diabetic screening camps and telemedicine-based platforms. However, all of these require trained examiners or graders to examine patients or read fundus photos. This leads to bottlenecks in the screening process, making it difficult to broadly screen populations.
In the United States, there are currently 2 FDA-cleared artificial intelligence (AI) platforms approved for the screening and detection of diabetic retinopathy.8 Artificial intelligence uses deep learning to identify patterns. Deep learning is a computational method that allows the algorithm to modify itself by learning from a large set of examples.8 Artificial intelligence is widely used throughout other areas, including telecommunications and social media. The application to ophthalmology opens the door to help increase access to diabetic retinopathy patient screening. The AI platform, mobility of the camera, and ability to take photos without dilation allow for widespread use of the device without the need for highly skilled staff. This, in turn, can make screening more accessible and flexible for the diabetic population.
IDX-DR
The IDx-DR (Digital Diagnostics) is the first FDA-cleared artificial intelligence algorithm for the detection of diabetic retinopathy. It was cleared by the FDA in 2018.9 The device is paired with a nonmydriatic Topcon fundus camera and the captured images are sent to a cloud-based server. The AI software then analyzes the images using a deep-learning algorithm to detect the presence or absence of diabetic retinopathy, based on ETDRS level 35 or higher.9 Hence, the IDx-DR is configured to detect more than mild diabetic retinopathy (mtmDR).
In real time, the patient receives a diagnosis of “No Diabetic Retinopathy Detected” or “Diabetic Retinopathy Detected” (Figure 1). Images that cannot be analyzed by the system accurately due to media or lens opacity will list “Exam Quality Insufficient.” Based on the read from the camera, subsequent follow-up can be determined. The sensitivity and specificity of the camera to detect more than mild diabetic retinopathy is reported to be 87.4% and 89.5%, respectively.9 Patients with known history of retinopathy, persistent vision loss, blurred vision, floaters, previously diagnosed macular edema, or other forms of retinal vascular disease should not be screened with the camera. In addition, the camera is not meant to screen for retinopathy in patients who are pregnant.9
EYEART
The EyeArt system (Eyenuk) is the first FDA-cleared artificial intelligence platform to detect mtmDR and vtDR in 1 test (Figure 2). It was cleared by the FDA in 2020.10 It uses a cloud-based server to analyze images with deep learning, using Canon nonmydriatic fundus cameras. The device reports a 96% sensitivity and 88% specificity for detecting mtmDR and 92% sensitivity and 94% specificity for detecting vtDR.10 Patients get a real-time diagnosis at the time of imaging. Similar limitations exist for imaging patients, including visual impairment, history of retinopathy, history of other vascular disease, history of intraocular surgery (other than uncomplicated cataract surgery), or hypersensitivity to the light from fundus photographs.11
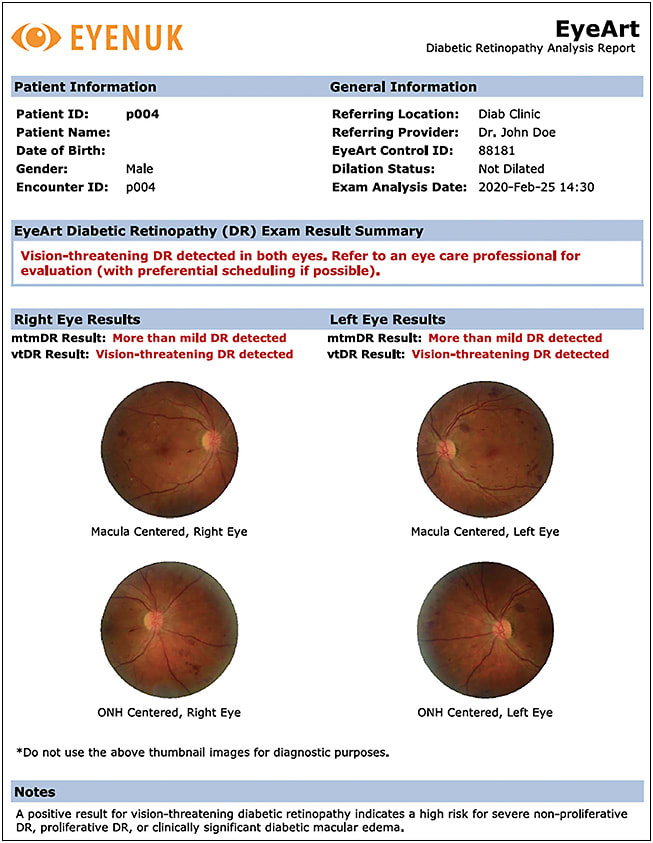
OTHER AI ALGORITHMS
There are other AI platforms in development or reported for screening of diabetic retinopathy, including by Retmarker, the Singapore Eye Research Institute, and Google.8
LIMITATIONS OF ARTIFICIAL INTELLIGENCE
There are certainly limitations to artificial intelligence in the screening of diabetic retinopathy. First, given the IDx-DR sensitivity and specificity of 87.4% and 89.5%, respectively, 1 in 10 patients will have a false-positive or false-negative result. Although the sensitivity and specificity is improved for the Eyenuk device, similar concerns apply. Furthermore, it remains to be seen whether the device can pick up subtle levels of macular edema in the absence of retinopathy. Lastly, the device does not screen for other ocular conditions, including glaucoma, cataracts, and macular degeneration, which are also highly prevalent. Therefore, a negative AI screen may generate a false sense of security for the patient, who then may not be motivated to get a dilated eye exam or intraocular pressure measurement to check for other common ocular conditions.
FINAL THOUGHTS
There is no doubt that there is an unmet need in diabetic retinopathy screening. With the increasing prevalence of diabetes and diabetic retinopathy, AI-based algorithms can be a welcome tool to help get patients into the health care system and screened for diabetic retinopathy. RP
REFERENCES
- Saeedi P, Petersohn I, Salpea P, et al. Global and regional diabetes prevalence estimates for 2019 and projections for 2030 and 2045: Results from the International Diabetes Federation Diabetes Atlas, 9th edition. Diabetes Res Clin Pract. 2019;157:107843. doi:10.1016/j.diabres.2019.107843
- Teo ZL, Tham YC, Yu M, et al. Global prevalence of diabetic retinopathy and projection of burden through 2045: systematic review and meta-analysis. Ophthalmology. 2021;128(11):1580-1591. doi:10.1016/j.ophtha.2021.04.027
- Chen AJ, Hwang V, Law PY, Stewart JM, Chao DL. Factors associated with non-compliance for diabetic retinopathy follow-up in an urban safety-net hospital. Ophthalmic Epidemiol. 2018;25(5-6):443-450. doi:10.1080/09286586.2018.1504311
- Puent BD, Nichols KK. Patients’ perspectives on noncompliance with diabetic retinopathy standard of care guidelines. Optometry. 2004;75(11):709-716. doi:10.1016/s1529-1839(04)70223-7
- Watane A, Kalavar M, Cavuoto KM, Sridhar J. Factors associated with follow-up non-compliance in patients presenting to an emergency department with non-proliferative diabetic retinopathy. Invest Ophthalmol Vis Sci. 2020;61(7):3831.
- Yannuzzi NA, Smiddy WE, Flynn HW Jr. Follow-up non-compliance: a significant risk factor for reduced visual outcomes in patients with diabetic retinopathy. Am J Ophthalmol. 2020;216:A12-A13. doi:10.1016/j.ajo.2020.04.004
- Liew G, Michaelides M, Bunce C. A comparison of the causes of blindness certifications in England and Wales in working age adults (16-64 years), 1999-2000 with 2009-2010. BMJ Open. 2014;4(2):e004015. doi:10.1136/bmjopen-2013-004015
- Grzybowski A, Brona P, Lim G, et al. Artificial intelligence for diabetic retinopathy screening: a review [published correction appears in Eye (Lond). 2019 Dec 10;:]. Eye (Lond). 2020;34(3):451-460. doi:10.1038/s41433-019-0566-0
- US Food and Drug Administration. FDA permits marketing of artificial intelligence-based device to detect certain diabetes-related eye problems. 2018. Accessed August 27, 2022. https://www.fda.gov/NewsEvents/Newsroom/PressAnnouncements/ucm604357.htm
- Eyenuk announces FDA clearance for EyeArt autonomous AI system for diabetic retinopathy screening. 2020. Accessed August 27, 2022. https://www.eyenuk.com/us-en/articles/diabetic-retinopathy/eyenuk-announces-eyeart-fda-clearance/
- Assessment of EyeArt as an automated diabetic retinopathy screening tool. Clinicaltrials.gov identifier: NCT03112005. Updated July 30, 2018. Accessed August 27, 2022. https://clinicaltrials.gov/ct2/show/NCT03112005