Ophthalmic imaging has advanced greatly since the last decade of the 20th century.1 Providing clinicians an objective tool to document, measure and evaluate disease progression, imaging has become an essential part of ophthalmic evaluation. Revolutionary imaging modalities such as optical coherence tomography (OCT) and ultrawidefield angiography have enabled assessment of the structures and details that are not observable by conventional ophthalmoscopy. The explosive growth in ophthalmic imaging has paralleled a dramatic increase in technology and the availability of computational power.2,3
Artificial intelligence (AI) is a broad term referring to the machines that mimic human cognitive functions such as problem-solving and learning. Machine learning is a type of AI with the ability to recognize meaningful patterns, and it has been widely applied in medical imaging. The introduction of deep convolutional neural networks (CNN) that can model complex nonlinear relationships has revolutionized computer vision and has the potential to lead to huge advances in medical image computation. Deep learning has impacted several fields of medicine with various health care applications including computer-aided detection, diagnosis, risk assessment, survival analysis, efficient care delivery, and drug discovery.4 Ophthalmology is one of the fields that is actively exploring the role of these new image analysis platforms and techniques that have emerged through advances in computer vision.
In retinal disease, the identification of imaging biomarkers is promising not only for expanding the understanding of pathophysiology but also for introducing new possibilities for personalized treatments. Furthermore, studies have demonstrated the potential of ophthalmic imaging biomarkers in early diagnosis of neurologic conditions.5-7 Machine learning systems have emerged as useful tools in image analysis and characterization. Current applications of machine learning for imaging biomarker assessment include disease identification, disease characterization, segmentation of regions of interests (ROI), and meaningful feature identification. Manual segmentation, which is accepted as ground truth, is very labor intensive and not always feasible. Another disadvantage of manual segmentation is inter-reader and intrareader variability.8 Semiautomated and automated segmentation tools created by machine learning algorithms are emerging to address these concerns. Also, machine learning techniques are facilitating selection of appropriate features because of their superiority in analysis of complex data sets.8 In addition to traditional machine learning and AI-based image interpretation, radiomics is an emerging area of research that is investigating numerous subvisual features of images and their associations with specific clinical data. The theory of radiomics suggests that signals for different pathophysiology that may not be identified by naked eye are present in images. Radiomics analysis can extract the signals that may be useful to differentiate distinct forms of the diseases, diagnose diseases at earlier stages, and predict prognosis and response to treatment.9
Hundreds of different radiomic features with various complexity levels can be extracted from a single image. The most basic are semantic features that are used commonly in clinic. These include geometric features like shape, volume, diameter, and maximum distance. More complex radiomic features are extracted from original or processed images by computational algorithms. They are categorized into first-order statistics features and second-order statistics.8 First-order statistics features evaluate voxels individually and ignores their spatial location and relationships. Features extracted by first-order statistics include mean, median, maximum, minimum, asymmetry, uniformity, and randomness (entropy) of voxel intensities. Second-order statistical features evaluate the voxels and take their spatial arrangement into account. They measure intralesion heterogeneity and are commonly referred as textural features.8 This article will focus on AI applications and identified biomarkers in ophthalmic imaging modalities.
FUNDUS PHOTOGRAPHY
Direct visualization of retinal vessels with biomicroscopy and fundus photography enables physicians to detect early manifestations of chronic illnesses, such as diabetes mellitus, hypertension, and other cardiovascular disorders.10 Machine learning applications in fundus photography have been used to identify retinal biomarkers for risk stratification and prognosis in chronic diseases.2,6 Color fundus images have been shown to include adequate information to predict risk factors of cardiovascular diseases such as age, blood pressure, smoking status, and HbA1c level.2 Deep learning attention maps have indicated that vascular features are mainly dictating these predictions.2 This finding is consistent with the use of vascular features, such as caliber, tortuosity, and microvascular changes, in routine care to diagnose and follow ophthalmic involvement of systemic conditions. Specifically, vessel diameter variations are demonstrated to be associated with cardiovascular risk.6 Arteriolar narrowing and venular widening have been associated with hypertension, diabetes, dyslipidemia, increased risk of stroke, and cardiovascular death. Venular widening has also been found to be associated with vascular dementia and systemic inflammation.6 Interestingly, in addition to vascular features, macular region of fundus photographs is predictive of cardiovascular risk by differentiating gender.2
Utilizing fundus photography, the first-ever FDA-cleared autonomous AI tool is now available. IDx-DR (IDx Technologies) screens and diagnoses diabetic retinopathy (DR) using color fundus photos. The platform analyzes the images within minutes and suggests retesting in a year or referral to an ophthalmologist based on DR status. The system has a sensitivity of 87% and specificity of 90% at detecting more than mild DR.
OPTICAL COHERENCE TOMOGRAPHY
Optical coherence tomography has the advantage of 3-dimensional visualization of retinal layers at micrometer resolution. Therefore, it has become a critical part of diagnosis and management in routine ophthalmologic care. With the increasing prevalence of retinal disorders, such as age-related macular degeneration (AMD) and diabetic eye disease, more than 30 million OCT scans are performed annually.3 Given the widespread utilization for retinal disease and the high resolution volumetric information, OCT has become an emerging modality for the exploration of imaging biomarkers through computational and deep-learning techniques. Generally, OCT research has focused on 2 main focuses: (1) enhanced automated segmentation of known retinal features (Figure 1) and (2) imaging biomarker discovery for diagnosis and characterization of ophthalmic and nonophthalmic diseases. One of the early commercial applications of AI to OCT was led by DeepMind Technologies Limited, an Alphabet-owned company that provides pioneering AI solutions. This diagnosis and referral tool was trained on more than 14,800 OCT scans.11 This AI-based platform can identify more than 50 ophthalmic conditions and recommend expert referral when necessary.
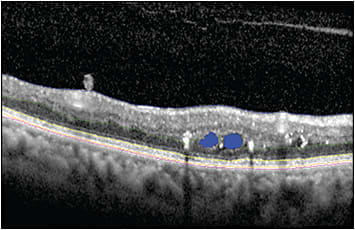
Disease activity biomarkers that are readily identified by visual inspection, such as subretinal fluid, intraretinal fluid, drusen, and geographic atrophy (GA), play a central role in current ophthalmic care and disease management. Various retinal layer integrity features have been proven to be useful diagnostically and for prognostication in DR, AMD, vitreomacular traction, and retinal dystrophies. Increased central subfield thickness and decreased retinal nerve fiber layer and ganglion cell layer have been associated with increased severity of DR.12 Furthermore, disruption of retinal inner layers (DRIL) in foveal and parafoveal areas are shown to be predictive for worse visual acuity in DR patients.12 In nonexudative AMD, decrease in drusen volume, outer retinal thinning, and presence of hyper-reflective foci are associated with the development of GA.12,13 In exudative AMD, the presence of intraretinal fluid has been associated with worse visual outcomes,12 both DRIL and outer retinal disruption (eg, ellipsoid zone and external limiting membrane loss), have been predictive of visual acuity in multiple diseases including DR, retinal vein occlusion, AMD, inherited retinal dystrophies, and hydroxychloroquine toxicity (Figure 2).12,14-16
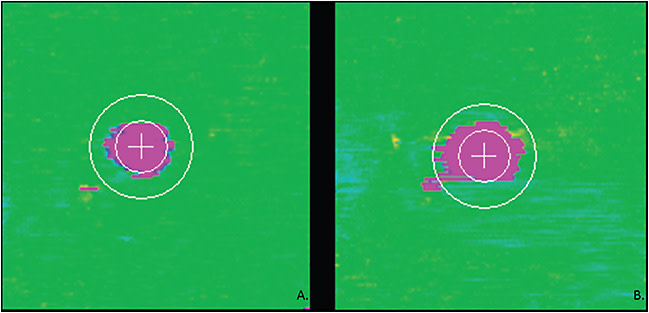
More in-depth image assessment and computational analysis of OCT features may lead to additional biomarkers and automated detection of known biomarkers that may guide disease understanding and therapeutic decision-making. Higher-order radiomics analysis, such as textural analysis of drusen, may provide unique insights into prognostic disease features. For example, heterogeneous internal hyper-reflectivity of drusenoid lesions and hyper-reflective foci located over drusen are found to be predictive for GA.12 Similarly, hyper-reflective foci located in outer retinal layers and spatial relation of ellipsoid zone and external limiting membrane are found to be prognostic biomarkers of visual acuity in diabetic macular edema.12,17 The ability to quantify these sub-RPE features would enable new exploratory assessments of prognostication and possible insights for future therapeutics. Similarly, optical density/reflectivity of subretinal fluid has been identified as a potential important biomarker in multiple disorders. Studies have demonstrated that increased subretinal fluid reflectivity compared to that of vitreous is associated with blood–retinal barrier function and anti-VEGF response. In addition, changes in the optical density of subretinal fluid has been shown to be correlated with visual acuity changes in exudative AMD patients.12 Textural analysis studies investigating subretinal and intraretinal fluids for prognostic biomarkers for treatment response are being actively explored.
Retinal imaging characteristics on OCT are also being examined as potential biomarkers of various nonophthalmic diseases. Subclinical and clinically apparent structural changes in retina have been implicated as indicators of various neurodegenerative and neuropsychiatric diseases.7 The potential utility of an OCT-based disease surveillance tool could be a significant advance in the screening for many of these conditions given its rapid noninvasive nature and low acquisition cost. Although the current research is not definitive, numerous OCT parameters and features have been described in association with neurodegenerative disorders. Thinning of the macular ganglion cell layer and inner plexiform layer has been associated with cognitive impairment, schizophrenia, and bipolar disorders.5,7,18 A reduced number of retinal ganglion cells resulting in thinner retinal nerve fiber layer and ganglion cell complex has been correlated with cerebral amyloid burden in Alzheimer disease.5 Photoreceptor outer segment integrity/volume, as measured by ellipsoid zone mapping, has been shown to be correlated with cognitive testing results in Alzheimer disease and Parkinson disease.19 In addition, a recent textural analysis of retinal layers at the macula differentiated between Alzheimer disease, Parkinson disease, and healthy controls, even in the absence of detectable differences in thickness measurements.20 Multicenter studies investigating higher-order radiomic features are needed to better assess the potential of retinal biomarkers in neurodegenerative diseases.
OPTICAL COHERENCE TOMOGRAPHY ANGIOGRAPHY
Optical coherence tomography angiography (OCTA) is a relatively new noninvasive method to image retinal and choroidal vessel detail. There are numerous commercially available OCTA devices, including from Zeiss, Optovue, Topcon, and Heidelberg. OCTA enables depth-encoded visualization of the capillary meshwork in significant detail, introducing new imaging biomarkers such as vascular density and foveal avascular zone. Microvasculature visualization also enables more complex radiomic feature analysis regarding vascular complexity. Fractal dimension (ie, complexity of vessel branching pattern) and lacunarity (ie, heterogeneity of vessels structure) are shown to be decreased in DR and retinal vein occlusion.21,22 One study demonstrated an association between lacunarity and vessel density measures of central macular deep capillary plexus on OCTA with peripheral nonperfusion on fluorescein angiography.21 In addition to local disease features, preliminary studies have suggested that detection of micron-level abnormalities of microvasculature makes OCTA a potential alternative to diagnose neurodegenerative diseases at subclinical stages, similar to OCT.23
ULTRAWIDEFIELD FLUORESCEIN ANGIOGRAPHY
Ultrawidefield fluorescein angiography (UWFA) provides near-panretinal assessment of the retinal vascular features of the eye. This modality enables visualization of specific vascular features that may enhance understanding of disease burden and optimize diagnostic accuracy. Areas of nonperfusion (eg, ischemia), vascular leakage, microaneurysm detection, and neovascularization are among known clinically apparent biomarkers that assist diagnosis, choice of treatment, and assessment of treatment response. Historically, rapid quantification of these features and objective severity scales have not been feasible. Emerging segmentation technology, including machine learning-enabled platforms, now provide the opportunity for automated quantification of multiple UWFA parameters (Figure 3). As an example, leakage index, ischemic index, and microaneurysm counts are demonstrated to correlate strongly with clinical severity of DR.24 More complex features, such as complexity of branching pattern of vessels, are also potential biomarkers for more objective grading scales. As an example, peripheral retinal vessels of diabetic eyes have been demonstrated to have lower complexity in their branching pattern compared to healthy controls.25 Radiomics-based tortuosity measures have been associated with therapeutic durability of anti-VEGF therapy in retinal vascular diseases.26,27
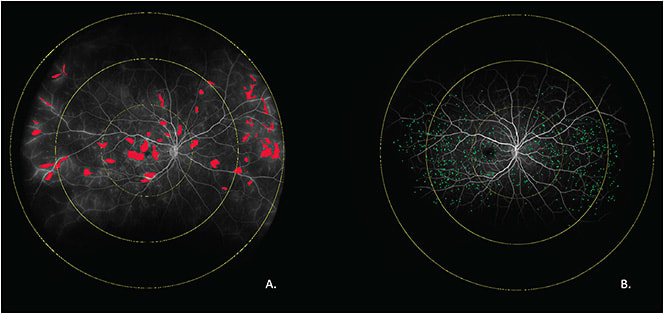
MULTISPECTRAL AND HYPERSPECTRAL RETINAL IMAGING
Spectrometry research in retina has mainly focused on ocular oximetry and early detection of nonperfused areas.28,29 Different stages of oxidative stress and degeneration in retinal ganglion cells have been demonstrated using the spectral characteristics of the oxidized state of cytochrome c.30 The results interpreted spectral imaging as a promising and rapid alternative method for early diagnosis of age related macular degeneration. Zilia Health has developed a research-based platform spectral oximetry with an AI-based image analysis platform for screening and assessment of retinal disorders, such as diabetic eye disease.
FUTURE DIRECTIONS
Applications of advanced computational image analysis systems and AI platforms are an exciting and emerging area of research in the field of imaging biomarkers. These new fields may lead to discovery of new patterns of disease, provide insights to pathophysiology, and facilitate precision medicine and individualized therapeutic decision-making. Larger studies investigating both the role of radiomics-based assessment and machine-learning platforms are needed to fully explore the potential of imaging biomarkers in predicting treatment outcomes and personalized treatment. RP
REFERENCES
- Bennett TJ, Barry CJ. Ophthalmic imaging today: an ophthalmic photographer’s viewpoint - a review. Clin Exp Ophthalmol. 2009;37(1):2-13.
- Poplin R, Varadarajan AV, Blumer K, et al. Prediction of cardiovascular risk factors from retinal fundus photographs via deep learning. Nat Biomed Eng. 2018;2(3):158-164.
- Swanson EA, Fujimoto JG. The ecosystem that powered the translation of OCT from fundamental research to clinical and commercial impact [invited]. Biomed Opt Express. 2017;8(3):1638-1664.
- Lundervold AS, Lundervold A. An overview of deep learning in medical imaging focusing on MRI. Z Med Phys. 2019;29(2):102-127.
- Cheung CY, Chan VTT, Mok VC, Chen C, Wong TY. Potential retinal biomarkers for dementia: what is new? Curr Opin Neurol. 2019;32(1):82-91.
- MacGillivray TJ, Trucco E, Cameron JR, Dhillon B, Houston JG, van Beek EJ. Retinal imaging as a source of biomarkers for diagnosis, characterization and prognosis of chronic illness or long-term conditions. Br J Radiol. 2014;87(1040):20130832.
- Nguyen CTO, Hui F, Charng J, et al. Retinal biomarkers provide “insight” into cortical pharmacology and disease. Pharmacol Ther. 2017;175:151-177.
- Gillies RJ, Kinahan PE, Hricak H. Radiomics: images are more than pictures, they are data. Radiology. 2016;278(2):563-577.
- Patyk M, Silicki J, Mazur R, Krecichwost R, Sokolowska-Dabek D, Zaleska-Dorobisz U. Radiomics - the value of the numbers in present and future radiology. Pol J Radiol. 2018;83:e171-e174.
- Cabrera DeBuc D, Somfai GM, Koller A. Retinal microvascular network alterations: potential biomarkers of cerebrovascular and neural diseases. Am J Physiol Heart Circ Physiol. 2017;312 (2):H201-H212.
- De Fauw J, Ledsam JR, Romera-Paredes B, et al. Clinically applicable deep learning for diagnosis and referral in retinal disease. Nat Med. 2018;24(9):1342-1350.
- Phadikar P, Saxena S, Ruia S, Lai TY, Meyer CH, Eliott D. The potential of spectral domain optical coherence tomography imaging based retinal biomarkers. Int J Retina Vitreous. 2017;3:1.
- Schmidt-Erfurth U, Waldstein SM. A paradigm shift in imaging biomarkers in neovascular age-related macular degeneration. Prog Retin Eye Res. 2016;50:1-24.
- Ugwuegbu O, Uchida A, Singh RP, et al. Quantitative assessment of outer retinal layers and ellipsoid zone mapping in hydroxychloroquine retinopathy. Br J Ophthalmol. 2019;103(1):3-7.
- Arepalli S, Traboulsi EI, Ehlers JP. Ellipsoid zone mapping and outer retinal assessment in Stargardt disease. Retina. 2018;38(7):1427-1431.
- Banaee T, Singh RP, Champ K, et al. Ellipsoid zone mapping parameters in retinal venous occlusive disease with associated macular edema. Ophthalmol Retina. 2018;2(8):836-841.
- Ehlers JP, Uchida A, Hu M, et al. Higher-order assessment of OCT in diabetic macular edema from the vista study: ellipsoid zone dynamics and the retinal fluid index. Ophthalmol Retina. 2019. [Epub ahead of print]
- Cabrera DeBuc D, Gaca-Wysocka M, Grzybowski A, Kanclerz P. Identification of retinal biomarkers in alzheimer’s disease using optical coherence tomography: recent insights, challenges, and opportunities. J Clin Med. 2019;8(7):E996.
- Uchida A, Pillai JA, Bermel R, et al. Outer retinal assessment using spectral-domain optical coherence tomography in patients with Alzheimer’s and Parkinson’s disease. Invest Ophthalmol Vis Sci. 2018;59(7):2768-2777.
- Nunes A, Silva G, Duque C, et al. Retinal texture biomarkers may help to discriminate between Alzheimer’s, Parkinson’s, and healthy controls. PLoS One. 2019;14(6):e0218826.
- Cabral D, Coscas F, Glacet-Bernard A, et al. Biomarkers of peripheral nonperfusion in retinal venous occlusions using optical coherence tomography angiography. Transl Vis Sci Technol. 2019;8(3):7.
- Zahid S, Dolz-Marco R, Freund KB, et al. Fractal dimensional analysis of optical coherence tomography angiography in eyes with diabetic retinopathy. Invest Ophthalmol Vis Sci. 2016;57(11):4940-4947.
- Mardin CY, Hosari S. [Optical coherence tomography angiography in neuronal diseases: preliminary findings]. Ophthalmologe. 2019;116(8):714-721.
- Ehlers JP, Jiang AC, Boss JD, et al. Quantitative ultra-widefield angiography and diabetic retinopathy severity: an assessment of panretinal leakage index, ischemic index and microaneurysm count. Ophthalmology. 2019;126(11):1527-1532.
- Fan W, Nittala MG, Fleming A, et al. Relationship between retinal fractal dimension and non-perfusion in diabetic retinopathy on ultra-wide field fluorescein angiography. Am J Ophthalmol. 2020;209:99-106.
- Moosavi A FN, Prasanna P, Srivastava SK, et al. Predicting tolerance to extended interval dosing in diabetic macular edema and retinal vein occlusion via subvisual feature assessment of ultra-widefield angiography: preliminary findings in the PERMEATE study. Presented at ARVO; 2019; Vancouver, Canada.
- Ehlers JP FN, Prasanna P, Hu M, et al. Advanced image analysis and machine-learning assessment of the impact of aflibercept on anatomic feature and flow dynamics in the PERMEATE study. Presented at ASRS Annual Meeting; 2018; Vancouver, Canada.
- Nourrit V, Denniss J, Muqit MM, et al. High-resolution hyperspectral imaging of the retina with a modified fundus camera. J Fr Ophtalmol. 2010;33(10):686-692.
- Gao L, Smith RT, Tkaczyk TS. Snapshot hyperspectral retinal camera with the image mapping spectrometer (IMS). Biomed Opt Express. 2012;3(1):48-54.
- Schweizer JH, Steiner G, Knels L, Funk R, Koch E. Hyperspectral imaging - a new modality for eye diagnostics. Biomedizinische Technik Biomed Eng. 2012.