Age-related macular degeneration (AMD) is the leading cause of blindness in people older than 50 years in developed countries and is among the top 3 most common causes of vision loss worldwide.1 Since AMD is mostly asymptomatic in its early stages, it is usually only diagnosed when patients seek routine eye examinations or are imaged with retinal color photography or optical coherence tomography (OCT). Of course, with limited treatment options for early AMD, early diagnosis has not been an issue. Nevertheless, investigators have been exploring the development of systemic biomarkers of AMD. Identifying plasma and/or urine biomarkers may help to identify subjects with AMD, correlate with disease progression, and offer the potential to provide personalized treatment for AMD. Metabolomics — the study of small molecules within a biological system — has the potential not only to advance our understanding of the mechanisms underlying AMD and other vitreoretinal diseases, but also to identify potential biofluid biomarkers of disease and disease progression.
METABOLOMICS FOR BIOMARKER IDENTIFICATION
Metabolomics is the qualitative and quantitative study of all small molecules <1.5 kDa, or metabolites, in a biological system. Metabolites are the downstream products of genetic transcription and translation, and they are affected by environmental exposures, including nutrition, lifestyle, and commensal organisms. In contrast to the genome, which remains relatively stable over a lifespan, the metabolome changes, representing a snapshot of a particular state and/or time. Of all the ’omics, the metabolome is thought to be the most closely linked to phenotype — providing information on normal and pathologic conditions, as well as the response to external stimuli. Metabolomics is a very powerful tool with great potential in clinical translation and precision medicine, and it has been investigated in complex conditions, including Alzheimer disease and cancer, as well as more recently in AMD.
BIOFLUIDS FOR SAMPLING
Currently, blood (serum and plasma) and urine are the 2 biofluids most commonly used in metabolomics. There is more experience using plasma, but urine has potential, as it is less expensive and easily accessible. Other biofluids that may be used in metabolomic studies include saliva, tears, and aqueous and vitreous humor (for ocular diseases). Although tissue samples may also be analyzed for investigational purposes, they are often preserved with fixative and embedded in paraffin for storage, which affects the metabolome.
Unlike the genetic sequence, the metabolomic profile varies, depending on the biofluid that is sampled. Therefore, appropriate sample collection and processing is important. As many metabolites have very rapid turnover rates, it is critical to handle samples as quickly as possible to prevent metabolite degradation. All efforts should be made to develop standardized protocols for collection and analysis to ensure uniformity within the study.
SAMPLE ANALYSIS
Two main analytical tools are used in metabolomics: nuclear magnetic resonance (NMR) spectroscopy and mass spectrometry (MS).2,3 Depending on the instrumentation and protocol used, these platforms can identify 50 to 5,000 different metabolites in a single analysis. However, no current tool, metric, or platform is able to identify all existing metabolites in a single run. Similar to biofluid samples, consideration should be given to the choice of technical analytical tool, as most metabolomic studies use a single analytical source. NMR spectroscopy offers the advantages of minimal sample preparation, the ability to reuse samples, high reproducibility, and less susceptibility to instrument variability. NMR is not as sensitive as MS, and interpretation of NMR spectra results is complex and requires substantial training. Although MS requires complex sample preparation, it can detect a broader range and increased number of metabolites compared to NMR, and more investigators are moving to MS analysis.
METABOLOMICS AND AMD
Metabolomics has the potential to advance our understanding of the underlying mechanisms and help us find novel biomarkers of AMD. Researchers have identified possible AMD biomarkers by comparing the metabolomics profiles of plasma samples from patients with and without neovascular AMD. Using liquid chromatography mass spectrometry (LC-MS), Osborn et al first described 94 metabolites that were significantly different between control subjects (n=19) and patients with AMD (n=26) — with 86 of the metabolites identified as known metabolites.4 Of the 94 metabolites identified, 40 were consistently different between the 2 groups. Pathway analysis of the identified metabolites mapped to 17 pathways mostly related to carbohydrate, amino acid, and coenzyme metabolites that are required for nitrogen balance and energy metabolism. In a Chinese patient population (20 patients with AMD, 20 controls), Luo et al also found differences in metabolic profiles and pathways between patients with and without neovascular AMD.5 They identified 10 significant metabolites, most of which were involved in amino acid metabolism.
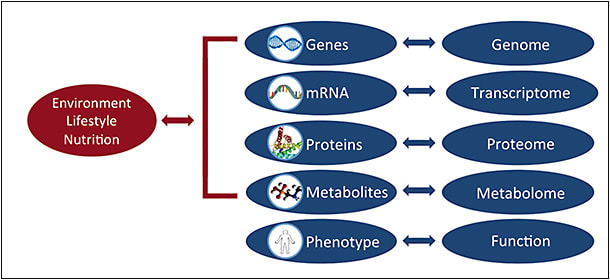
Recently, our group at Mass. Eye and Ear described differences in metabolomic profiles between patients with AMD and controls, as well as metabolites in patients with AMD across different stages of the disease (early, intermediate, late). Our initial work included NMR analysis of 396 total subjects from 2 different study sites — Coimbra, Portugal (n=243; 42 controls and 201 patients with AMD), and Boston, Massachusetts (n=153; 40 controls and 113 patients with AMD).6 An independent analysis of the 2 cohorts identified a separation between multiple AMD stages for the Boston cohort, and between extreme AMD stages (late AMD vs controls; late AMD vs early AMD) for the Coimbra cohort. Interestingly, metabolites were identified in each cohort as significantly different between control subjects and patients diagnosed with early AMD — acetate, creatine, dimethyl sulfone, cholesterol, HDL-choline, and unsaturated fatty acids for the Coimbra cohort; albumin, histidine, glutamine, and unsaturated fatty acids for the Boston cohort. Unsaturated fatty acids were common to both the Coimbra and Boston cohorts.
In a second study, we conducted MS analysis to measure a broader range of metabolites in patients with and without AMD. In the Boston cohort, the metabolomics profile was characterized for 90 total patients with AMD — 30 with early AMD, 30 with intermediate AMD, and 30 with late AMD — and 30 controls.7 After controlling for age, gender, body mass index, and smoking status, 87 metabolites differed significantly between patients with and without AMD. Of these metabolites, more than half (48 metabolites) differed significantly across AMD severity stages. Similar to the work of Osborn et al and our NMR study, we identified significantly increased levels of dipeptides and amino acid metabolites in patients with AMD, including a significant role for alanine and aspartate metabolism. Interestingly, according to our MS analysis, the majority of the identified significant metabolites were involved in lipid metabolism, specifically glycerophospholipid metabolism. Glycerophospholipids, which are a major component of cell membranes, are especially enriched in neural membranes and have been a focus of investigation in neurodegeneration and a number of chronic neurologic diseases.8,9 While these findings are promising, we are currently performing additional studies with the Boston and Coimbra cohorts to validate our results.
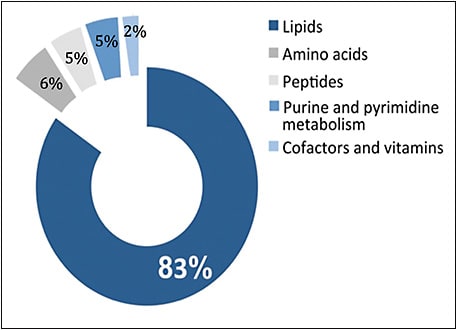
LIMITATIONS
As with all studies, there are important limitations to consider. The clinical applicability of metabolomics is strongly linked to the quality and accuracy of the data obtained. As such, study design should be planned prior to initiation to ensure high-quality data collection and low analytical variability. Important considerations include subject and sample selection, sample collection, size, handling, storage, and preparation, and data analysis. Additionally, many previous studies involving metabolomics are limited by poor design, lack of statistical power, and validated cohorts. Finally, metabolomics represents one approach to develop a useful biomarker for AMD. Other potentially useful biomarkers may be identified using proteomics, microbiome studies, or analysis of retinal imaging.
FUTURE DIRECTIONS
The field of metabolomics is rapidly expanding and holds great promise to significantly improve diagnosis, provide prognostic guidance, and identify potential therapeutic targets. While the application of metabolomics to the study of vitreoretinal diseases, including AMD, is in its early stages, the work from our group and others indicates that this novel tool has the potential to expand our knowledge of the pathogenesis of vitreoretinal diseases, and to identify diagnostic and prognostic biomarkers of disease. By contributing to better diagnostics, patient selection, treatment, and cost-effectiveness of treatment strategies, metabolomics has the potential to improve patient outcomes. Combining metabolomic profile data with imaging biomarkers, genomic profiling, and functional testing may lead to identification of AMD subtypes and ultimately personalized treatment options, heralding the era of precision medicine for AMD. RP
REFERENCES
- Wong WL, Su X, Li X, et al. Global prevalence of age-related macular degeneration and disease burden projection for 2020 and 2040: a systematic review and meta-analysis. Lancet Glob Health. 2014;2(2):e106-e116.
- Emwas AH. The strengths and weaknesses of NMR spectroscopy and mass spectrometry with particular focus on metabolomics research. Methods Mol Biol. 2015;1277:161-193.
- Barnes S, Benton HP, Casazza K, et al. Training in metabolomics research. I. Designing the experiment, collecting and extracting samples and generating metabolomics data. J Mass Spectrom. 2016;51(7):461-475.
- Osborn MP, Park Y, Parks MB, et al. Metabolome-wide association study of neovascular age-related macular degeneration. PLoS One. 2013;8(8):e72737.
- Luo D, Deng T, Yuan W, Deng H, Jin M. Plasma metabolomic study in Chinese patients with wet age-related macular degeneration. BMC Ophthalmol. 2017;17(1):165.
- Laíns I, Duarte D, Barros AS, et al. Human plasma metabolomics in age-related macular degeneration (AMD) using nuclear magnetic resonance spectroscopy. PLoS One. 2017;12(5):e0177749.
- Laíns I, Kelly RS, Miller JB, et al. Human plasma metabolomics study across all stages of age-related macular degeneration identifies potential lipid biomarkers. Ophthalmology. 2018;125(2):245-254.
- Farooqui AA, Horrocks LA, Farooqui T. Glycerophospholipids in brain: their metabolism, incorporation into membranes, functions, and involvement in neurological disorders. Chem Phys Lipids. 2000;106(1):1-29.
- Frisardi V, Panza F, Seripa D, Farooqui T, Farooqui AA. Glycerophospholipids and glycerophospholipid-derived lipid mediators: a complex meshwork in Alzheimer’s disease pathology. Prog Lipid Res. 2011;50(4):313-330.