Crowdsourcing the Analysis of Retinal Imaging Data
New technologies could facilitate diagnosis.
CHRISTOPHER J. BRADY, MD
Artificial intelligence. Big data. Computer vision. Crowdsourcing. Many new terms and concepts have entered the business and technology lexicon in recent years. Some of these technologies may fall by the wayside as mere “buzz words,” but some have the potential to bring significant changes to how we practice medicine in the coming years.
For example, Google (Mountain View, CA) was able for several years to predict trends in influenza outbreaks more rapidly than the Centers for Disease Control and Prevention by analyzing when and where users searched for flu-related symptoms.1 Computer vision and predictive algorithms are frequently in the press now in discussions about self-driving vehicles, which have the potential to revolutionize transportation in this country (particularly for those with visual disabilities).
So where are we in retina? Are we able to leverage all these breakthroughs? I would submit that the answer is “not quite.” I believe we are on the brink of dramatic improvements in the ways we diagnose and manage our patients, but the state of the art still relies on good old-fashioned human intelligence.
Perhaps it is this interface between human intelligence and artificial intelligence that might help to ease this transition to the widespread adoption of these new technologies. Crowdsourcing is a novel tool for innovation and data management that sits at this intersection and that may be helpful in bridging this gap.
Christopher J. Brady, MD, is assistant professor of ophthalmology at the Wilmer Eye Institute of Johns Hopkins University in Baltimore, MD. He reports no financial interests in products mentioned in this article. Dr. Brady can be reached via e-mail at brady@jhmi.edu.
CROWDSOURCING BACKGROUND
Daren Brabham, a new media scholar at the University of Southern California, defined crowdsourcing as “an online, distributed problem-solving and production model that leverages the collective intelligence of online communities to serve specific organizational goals.”2 He described four types of crowdsourcing, two of which are perhaps most relevant to biomedical research.2,3
First, “distributed-human-intelligence tasking” can involve subdividing larger tasks into small portions and then recruiting a group of individuals to complete each of these small portions and, only collectively, the entire task.
Second, a “broadcast search” or “innovation contest” involves the defining of a circumscribed empiric problem and promoting it “to an online community in the hopes that at least one person in the community may know the answer.”3
These two forms of crowdsourcing can be seen as opposite ends of the spectrum: the former involves recruiting a “swarm” to solve a small, simple problem collectively, and the latter involves finding a solitary expert to solve a very difficult problem; however, they share the concept of identifying solutions outside an organization that are not readily solvable by the organization itself, and both have been applied to research involving the retina.
Fundus Image Grading
Numerous groups are at work on automated fundus image analysis to determine the presence or absence of disease and, ultimately, the degree thereof. Despite these efforts, there is currently no FDA-approved artificial intelligence program for fundus photo grading, creating a possible space for crowdsourcing.
Danny Mitry and colleagues, at Moorfields Eye Hospital in London, reported an experiment using the Amazon.com (Seattle, WA) distributed human intelligence crowdsourcing marketplace, Amazon Mechanical Turk (AMT), to determine whether unskilled Web users could differentiate normal fundus images from those with a variety of pathological features.4 The investigators found a sensitivity of ≥96% for severely abnormal findings and between 61% and 79% for mildly abnormal findings.
Brady and colleagues explored whether untrained users of AMT would be able to reliably detect varying levels of diabetic retinopathy.5 For this study, users could review several training images describing a normal fundus and the various pathological features of DR. On the same page, the users were presented with one of 19 teaching images and were asked to grade the fundus as normal or abnormal (Figure 1).
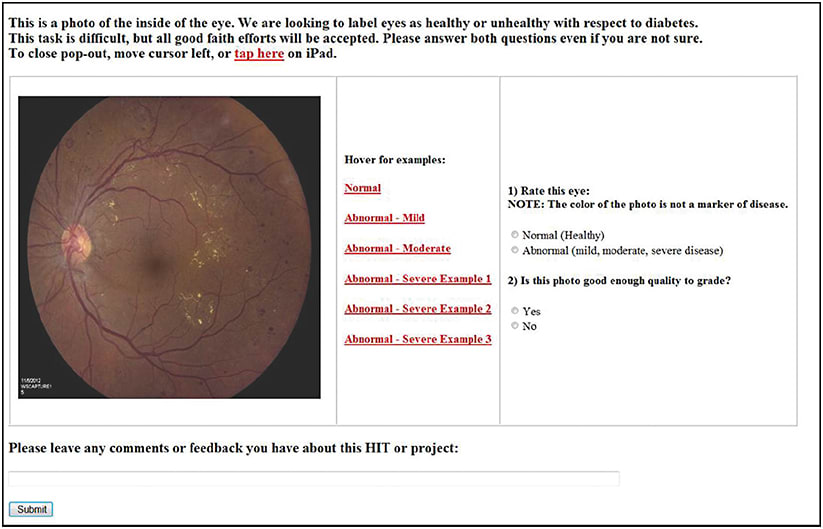
Figure 1. A screen capture of the Amazon Mechanical Turk crowdsourcing interface used by Brady et al.
The users spent an average of 25 seconds, including the time spent viewing the training images, and they were correct 81.3% of the time. Using feedback from the initial batches, the Web interface was improved, and user accuracy likewise improved, although user ability to grade the specific level of DR remained suboptimal.
A challenge of crowdsourcing is how to process the raw results into a single “score” for each image. A separate experiment by Brady confirmed that requesting 10 independent interpretations and using the average for the final “grade” was an appropriate strategy, maximizing the sensitivity and specificity, with minimal gains in either achieved by incorporating additional users’ grades.
When the same group of investigators asked AMT users to grade images from a much larger public dataset with more subtle disease,6,7 the users successfully identified images as abnormal when moderate to severe disease was present, but they were less successful at identifying very mild disease as abnormal (ie, ≤5 microaneurysms).8
Such experiments demonstrate that minimal training in retinal pathology and pattern recognition may be sufficient to identify clinically significant levels of disease. Because AMT is Web based, and users can be found in more than 65 countries (ie, in many time zones),9 a fully optimized crowdsourcing paradigm could provide near real-time image analysis for telemedicine programs.
Optical Coherence Tomography
A domain in which automated image processing has already made significant inroads in clinical ophthalmology practice is in the segmentation of retinal layers on optical coherence tomography images. Retinal nerve fiber layer measurements around the optic nerve and total retinal thickness measurements of the macular area are perhaps the most widely used segmentations, and numerous other layers and their thickness maps are likely to become increasingly valuable in the future.
Unfortunately, in pathologic states, which are obviously the most critical scans for clinicians, automated segmentations can become unreliable (Figure 2, page 40). Lee and colleagues presented their experience recruiting untrained AMT users to trace the layers of the retina on several markedly abnormal OCTs at the 2014 ARVO annual meeting.10
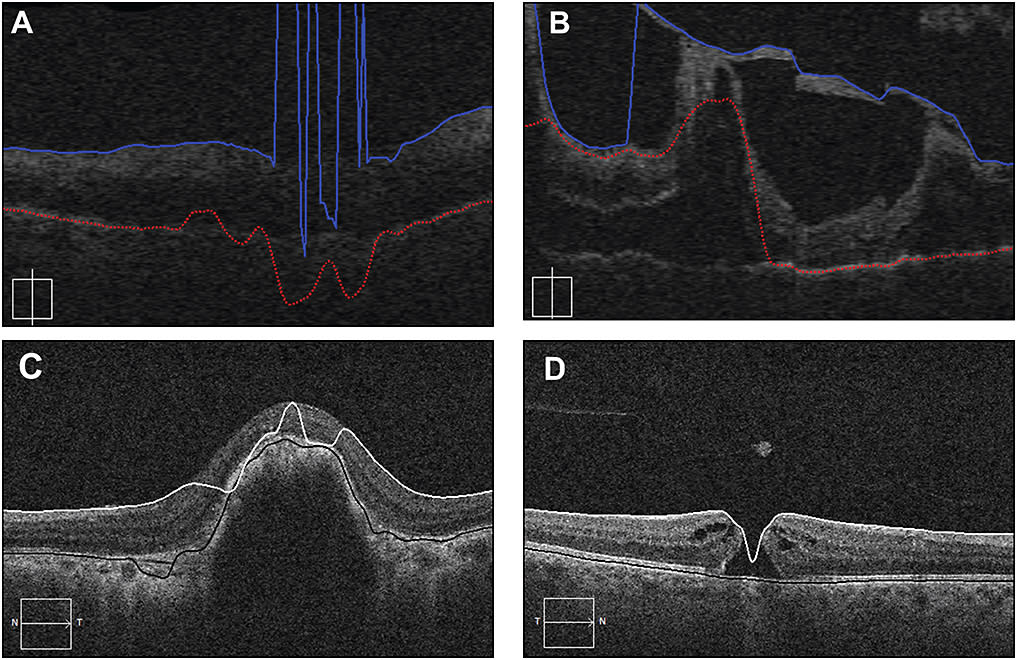
Figure 2. An assortment of OCT segmentation errors made by automated software. A) In this scan with poor signal strength centrally, the internal limiting membrane and retinal pigment epithelium are essentially completely lost. B) In this scan from diabetic tractional detachment, a thick posterior hyaloid tractional membrane is mistaken for the ILM and the algorithm loses the RPE on the left half of the scan. C) This patient with exudative AMD has a scan in which the ILM tracing jumps back and forth from the ILM to the RPE. This type of error will be propagated to the thickness map. D) In this patient with a full-thickness macular hole, the algorithm cannot detect the discontinuity of the ILM inherent in this disease.
This group found that they were able to generate reliable tracings at a very low cost. Each tracing took the workers an average of slightly more than 30 seconds, and it was performed for $0.01. The authors reported that the AMT workers succeeded specifically where the automated algorithms had failed.
Such an approach to OCT segmentation might be used to generate a wealth of “gold standard” or “ground truth” tracings to feed back into artificial intelligence algorithms so that the next generation of automated software can overcome its current shortcomings.
CROWDSOURCING IN BASIC SCIENCE: EYEWIRE
Crowdsourcing has also been deployed in several basic science undertakings, one of which perhaps truly embodies the promise of crowdsourcing. The EyeWire project was started by scientists at the Massachusetts Institute of Technology, and it has incorporated data generated by tens of thousands of Web users.11
The investigators set out to map individual ganglion cell neurons within the retina to better characterize poorly understood phenomena, such as the perception of motion. It turns out that creating a three-dimensional map of the branching patterns of mouse retinal neurons was extremely complicated and labor-intensive, even for the investigators’ dedicated laboratory staff. The investigators therefore decided to see whether transforming the task into a Web-based game could rapidly produce accurate maps.
To incentivize accuracy, several features were programmed into the game, such as a leaderboard that published the users’ high scores. Additionally, users could vote on a “consensus” segmentation, which significantly increased accuracy. This robust form of consensus allowed the investigators to take advantage of what New York Times business columnist James Surowiecki termed the “wisdom of crowds,”12 which can be more nuanced and sophisticated than merely using the arithmetic mean of different users’ responses to the same question.
When analyzing the EyeWire results, Kim and colleagues found that users made substantial commitments to the game, with the most accurate graders having logged thousands of neuron “cubes.” Interestingly, as evidence of the complexity of the task, workers continued to demonstrate improvements in accuracy over the course of dozens of hours of practice, in contrast with more rudimentary crowdsourcing tasks, which demonstrate a ceiling effect after minimal exposure to the task.
The investigators felt the contributions of the EyeWirers were substantial enough to warrant authorship on one of their manuscripts.11 This engagement has been termed “Virtual Citizen Science”13 and has been demonstrated to be a powerful motivator for people to participate in these types of tasks without financial rewards, as is used on the AMT interface. Crowdsourcers will work for free if the task is fun: they are contributing to an important project, and they can learn something by participating.
Because EyeWire develops highly skilled individuals and takes advantage of the emergent wisdom of the crowd, it is a distributed human intelligence task that shares some features of a broadcast search. The pure broadcast search is exemplified by the competitions hosted on kaggle.com. This Web site advertises itself as “The Home of Data Science” and describes itself as “a platform for predictive modelling and analytics competitions on which companies and researchers post their data and statisticians and data miners from all over the world compete to produce the best models.”14
As of early 2015, there is a competition to develop an artificial intelligence algorithm to interpret fundus photographs acquired during real-world DR telemedicine screening programs.15 The sponsoring company has posted more than 30,000 graded images that can be used to “train” the algorithm, as well as more than 50,000 images to be graded for the competition.
While there are options to find and recruit collaborators and merge data science teams, ultimately one team will win a grand prize of $50,000, and the sponsoring company will have access to the algorithm. As of March 11, 2015, with several months left to go, 119 teams have entered the contest and have submitted a total of 440 gradings.
CONCLUSIONS
It seems clear that automation will continue in the biomedical sciences, and in particular, there will be more automated image analysis in vitreoretinal medicine. Until this moment arrives, there is still an unmet need that can be partially filled with human intelligence. In fact, it may be human intelligence that helps to facilitate the arrival of fully automated image analysis by providing correctly interpreted images for the algorithms from which to “learn.”
A good example of this type feedback loop is the ReCAPTCHA system developed by Luis von Ahn and colleagues.16 ReCAPTCHA is the technology that generates the small boxes featuring distorted text that are often featured on the login pages of Web sites. To prove you are a legitimate user (ie, human), you must type in the letters or numbers in the box, and only then can you proceed to the Web site.
After Google purchased this technology, it began to submit words that had failed at least two optical character recognition algorithms and then the results back into the software. In addition, this project had the added benefit of digitizing difficult words of ancient texts for the Google Books library.
Indeed, EyeWire neuron segmentation results are similarly being used to improve artificial intelligence algorithms, and it is not difficult to imagine human-traced OCT retinal layer segmentations from AMT being fed into an automated algorithm, ultimately resulting a better-automated algorithm. The NIH feels there is promise in crowdsourcing in biomedical research, and it recently released a call for proposals using crowdsourcing.
Even in areas in which automated analyses seemed poised to take over from traditional methods of analyses, there is still room for human intelligence. Google flu trends have been improved upon in an iterative fashion by incorporating traditional surveillance data,1,17 suggesting that, perhaps even as automated image analysis improves, there may still be a need for human interaction. This has largely been the case with measurements of retinal vessel caliber with even the most state-of-the-art programs remaining “semi-”automated.18
This may be the strongest argument for continuing research in the crowdsourcing of retinal imaging data analysis; artificial intelligence and human intelligence may prove to be complementary technologies further into the future than it may currently appear. RP
REFERENCES
1. Davidson MW, Haim DA, Radin JM. Using networks to combine “big data” and traditional surveillance to improve influenza predictions. Sci Rep. 2015;5:8154.
2. Brabham DC. Crowdsourcing. The MIT Press Essential Knowledge Series. Cambridge, MA; MIT Press; 2013.
3. Brabham DC, Ribisl KM, Kirchner TR, Bernhardt JM. Crowdsourcing applications for public health. Am J Prev Med. 2014;46:179-187.
4. Mitry D, Peto T, Hayat S, Morgan JE, Khaw KT, Foster PJ. Crowdsourcing as a novel technique for retinal fundus photography classification: analysis of images in the EPIC Norfolk cohort on behalf of the UK Biobank Eye and Vision Consortium. PLoS One. 2013;8:e71154.
5. Brady CJ, Villanti AC, Pearson JL, Kirchner TR, Gupta OP, Shah C. Rapid grading of fundus photographs for diabetic retinopathy using crowdsourcing. J Med Internet Res. 2014;16:e233.
6. Sánchez CI, Niemeijer M, Dumitrescu AV, et al. Evaluation of a computer-aided diagnosis system for diabetic retinopathy screening on public data. Invest Ophthalmol Vis Sci. 2011;52:4866-4871.
7. MESSIDOR: Methods to evaluate segmentation and indexing techniques in the field of retinal ophthalmology. MESSIDOR Web site. Available at: http://messidor.crihan.fr/index-en.php. Accessed March 1, 2015.
8. Brady CJ. Rapid grading of fundus photos for diabetic retinopathy using crowdsourcing: refinement and external validation. Paper presented at: 47th annual scientific meeting of the Retina Society; Philadelphia, PA; September 13, 2014.
9. Ipeirotis PG. Demographics of mechanical turk. (CeDER Working Paper-10-01). New York University. New York, NY; 2010.
10. Lee AY, Tufail A. Mechanical Turk based system for macular OCT segmentation. Invest Ophthalmol Vis Sci. 2014;55:ARVO E-Abstract 4787.
11. Kim JS, Greene MJ, Zlateski A, et al; Eyewirers. Space-time wiring specificity supports direction selectivity in the retina. Nature. 2014;509:331-336.
12. Surowiecki J. The Wisdom of Crowds. New York, NY; Anchor Books; 2005.
13. Reed J, Raddick MJ, Lardner A, Carney K. An exploratory factor analysis of motivations for participating in Zooniverse, a collection of virtual citizen science projects. Paper presented at: 46th Hawaii International Conference on System Sciences (HICSS). Wailea, HI; January 7-10, 2013.
14. Kaggle: The Home of Data Science. Kaggle Web site. Available at: http://www.kaggle.com. Accessed March 9, 2015.
15. Identify signs of diabetic retinopathy in eye images. Diabetic Retinopathy Detection Kaggle Competition. Kaggle Web site. Available at: https://www.kaggle.com/c/diabetic-retinopathy-detection. Accessed March 11, 2015.
16. von Ahn L, Maurer B, McMillen C, Abraham D, Blum M. reCAPTCHA: Human-based character recognition via Web security measures. Science. 2008;321:1465-1468.
17. Butler D. When Google got flu wrong. Nature. 2013;494:155-156.
18. Cheung CY, Hsu W, Lee ML, et al. A new method to measure peripheral retinal vascular caliber over an extended area. Microcirculation. 2010;17:495-503.