Automated Choroidal Segmentation on OCT
A brief review of the techniques under investigation
JAY CHHABLANI, MD
Advances in optical coherence tomography have changed clinical practice over the last two decades, with OCT becoming an essential part of the management of macular diseases. Recently, imaging of the choroid has become possible with the advent of enhanced depth imaging (EDI), which has opened a new area of clinical research and improved our understanding of various chorioretinal diseases.1
So far, choroidal assessment on spectral-domain OCT has included measurement of choroidal thickness and quantitative evaluation of the choroidal vessel layer.2,3 Recent literature has reported changes in choroidal thickness in various diseases; however, this area still requires further exploration.4-7
These measurements are performed manually, which is a time-consuming, laborious procedure, with uncertain repeatability due to the subjective nature of image analysis, requiring training for the observers. Choroidal images are currently of low resolution with a high signal-to-noise ratio.
Therefore, the detection of the two boundaries that delineate the choroid is a complicated and challenging task, particularly the detection of the outer choroidal boundary due to the nonuniform nature of the vessels and their low contrast.
Over the years, significant improvement has occurred in acquisition speed and resolution, as well as in automated segmentation on retinal OCT. Automated segmentation of the retina provides various parameters, such as central macular thickness, retinal volume, and subfield thickness, which have become crucial parts of patient management and research.
However, automated segmentation of the choroid is still not available in clinical practice. Here, we describe various automated segmentation techniques, which are not yet commercially available, and their future prospects.
Jay Chhablani, MD, is on the faculty of the L. V. Prasad Eye Institute in Hyderabad, India. He reports no financial interests in any the products mentioned in this article. Dr. Chhablani can be reached via e-mail at jay.chhablani@gmail.com.
THE RESEARCH AT A GLANCE
Alonso-Caneiro et al reported an algorithm for segmentation of the inner and outer choroidal boundaries on SD-OCT images.8 The method used was a graph-searching theory to segment the two boundaries of interest. For inner boundary detection, the algorithm used an edge filter, while the outer boundary detection was based on dual brightness probability gradient information.
This method showed close agreement with manual analysis performed by an experienced observer. However, the algorithm takes a long time for a single B scan and has yet to be verified in various choroidal pathologies.
Tian et al found the choroidal boundary by finding the shortest path of the graph formed by valley pixels using dynamic programming.9 Danesh et al proposed a texture-based algorithm for fully automatic segmentation of choroidal images.10
Dynamic programming has been utilized to determine the location of the retinal pigment epithelium. Bruch’s membrane was segmented by searching for the pixels with the greatest gradient value below the RPE.
The choroid-sclera interface was segmented using wavelet-based features to construct a Gaussian mixture model. The main limitation of this method was the need for manual segmentation to construct the model.
Lu et al proposed a technique to segment the inner boundary of the choroid, using a two-stage fast active contour model.11 Then, real-time, human-supervised, automated segmentation of the outer boundary of the choroid was applied. The authors reported that the Dice similarity coefficient value of 30 images captured from patients diagnosed with diabetes was 92.7%.
Hu et al reported a semiautomated algorithm for choroidal segmentation using a graph-based, multistage, multisurface segmentation approach to segmenting the choroidal band — the layer between the outer RPE and choroid-sclera junction.12
An additional three surfaces — specifically the internal limiting membrane, inner-outer segment junction, and inner RPE surface — were also segmented to facilitate choroidal segmentation. The algorithm showed excellent agreement with human expert manual segmentation, particularly in the central portions of the volume scans.
Segmentation of individual choroidal vessels or layers has also been attempted. Due to the complexity and tightly packed nature of the choroidal vascular network, it is quite challenging to segment the choroidal vessels in each layer, particularly in the choriocapillaris, due to its very small size.
Zhang et al reported on automated choroidal vasculature thickness and choriocapillaris-equivalent thickness of the macula using three-dimensional SD-OCT.13 Choroidal thickness was defined as the thickness of the choroidal vasculature, ie, the upper surface of the vessel below Bruch’s membrane and the lower surface of the choroidal vessel above the sclera. Choriocapillaris thickness was measured between Bruch’s membrane and the upper surface of the choroidal vessel.
The reproducibility for segmentation was a coefficient of 0.78±0.08. However, the authors performed complex processing of C scans and did not compare their process with a manual technique.
Duan et al presented an automated algorithm to segment and quantitatively characterize the choroidal vessels on volumetric OCT obtained by high-penetration OCT.14 The choroidal vessels were segmented by thresholding-based segmentation, using an en face OCT slice at a constant relative depth to the RPE.
After segmentation, the thicknesses of the choroidal vessels were quantified by multiscale morphological analysis. The authors provided individual vascular network, Haller’s and Sattler’s layers, thickness maps, and distributions of the mean choroidal vessel diameter in nine different sectors in the macula.
Kajic et al reported choroidal vessel segmentation using a multiscale 3D edge filtering technique, followed by a statistical model using 1,060-nm 3D-OCT images.15 They reported the ratios of the inner (smaller) to outer (larger) choroidal vessels and the absolute/relative volume of the choroid vessels.
Statistical modeling provided robustness to the changes in signal-to-noise ratio and shape properties; therefore, it was useful even for OCT images with low ratios. This approach yielded good results (mean error of 13%) at the cost of requiring extensive training for the statistical model.
Our group reported an automated algorithm for individual choroidal vessel segmentation.16 The algorithm included denoising the image and contour detection. The correlation between the algorithm and manual segmentation was good. This algorithm provided choroidal vessel diameters and area, particularly of large and medium-sized vessels (Figure 1).
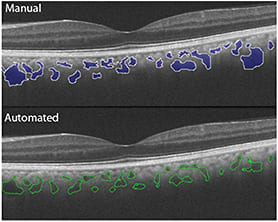
Figure 1. In this pair of images, manual segmentation (top) is compared with automated segmentation (bottom).
We also reported an automated algorithm for choriocapillaris segmentation on 2D-OCT images (Figure 2).17 Automated or manual segmentation of the choriocapillaris is very challenging due to its small size. Because of the limited transversal resolution of OCT images, segmentation of such small vessels, eg, the choriocapillaris, is unreliable, as noted in various reports.
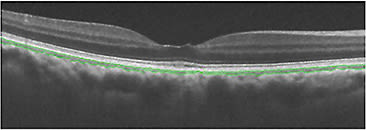
Figure 2. In this image, an automatic algorithm is used to segment the choriocapillaris (green) on a 2D image.
CONCLUSION
Automated segmentation of the choroid is now possible, providing choroidal thicknesses at various locations and choroidal volume at the macular region. However, assessment of individual vessels or of the vessel layer is still in research phase.
Various parameters, such as volumetric analysis, vessel diameter, light-dark ratio, vasculature thickness, and ratios of inner (smaller) to outer (larger) choroidal vessels, will become available for detailed choroidal assessment in the future. Evaluation of choroidal vessels, particularly the choriocapillaris, would constitute a great leap forward in posterior-segment imaging. Quantification of choroidal damage and more objective assessment would improve diagnostic ability and improve follow-up in various chorioretinal disorders. RP
REFERENCES
1. Spaide RF, Koizumi H, Pozzoni MC. Enhanced depth imaging spectral-domain optical coherence tomography. Am J Ophthalmol. 2008;146:496-500.
2. Chhablani J, Kozak IR, Mojana F, et al. Fundus autofluorescence not predictive of treatment response to intravitreal bevacizumab in exudative age-related macular degeneration. Retina. 2012;32:1465-1470.
3. Branchini LA, Adhi M, Regatieri CV, et al. Analysis of choroidal morphologic features and vasculature in healthy eyes using spectral-domain optical coherence tomography. Ophthalmology. 2013;120:1901-1908.
4. Jonas JB, Forster TM, Steinmetz P, et al. Choroidal thickness in age-related macular degeneration. Retina. 2013;34:1149-1155.
5. Chhablani J, Kozak I, Jonnadula GB, et al. Choroidal thickness in macular telangiectasia type 2. Retina. 2014 Jun 3 [Epub ahead of print]
6. Barteselli G, Lee SN, El-Emam S, et al. Macular choroidal volume variations in highly myopic eyes with myopic traction maculopathy and choroidal neovascularization. Retina. 2014;34:880-889.
7. Ayton LN, Guymer RH, Luu CD. Choroidal thickness profiles in retinitis pigmentosa. Clin Exp Ophthalmol. 2013;41:396-405.
8. Alonso-Caneiro D, Read SA, Collins MJ. Automatic segmentation of choroidal thickness in optical coherence tomography. Biomed Opt Express. 2013;4:2795-2812.
9. Tian J, Marziliano P, Baskaran M, et al. Automatic segmentation of the choroid in enhanced depth imaging optical coherence tomography images. Biomed Opt Express. 2013;4:397-411.
10. Danesh H, Kafieh R, Rabbani H, Hajizadeh F. Segmentation of choroidal boundary in enhanced depth imaging OCTs using a multiresolution texture based modeling in graph cuts. Comput Math Methods Med. 2014;2014:479268.
11. Lu H, Boonarpha N, Kwong MT, Zheng Y. Automated segmentation of the choroid in retinal optical coherence tomography images. Conf Proc IEEE Eng Med Biol Soc. 2013;2013:5869-5872.
12. Hu Z, Wu X, Ouyang Y, Sadda SR. Semiautomated segmentation of the choroid in spectral-domain optical coherence tomography volume scans. Invest Ophthalmol Vis Sci. 2013;54:1722-1729.
13. Zhang L, Lee K, Niemeijer M, et al. Automated segmentation of the choroid from clinical SD-OCT. Invest Ophthalmol Vis Sci. 2012;53:7510-7519.
14. Duan L, Hong YJ, Yasuno Y. Automated segmentation and characterization of choroidal vessels in high-penetration optical coherence tomography. Opt Express. 2013;21:15787-15808.
15. Kajic V, Esmaeelpour M, Povazay B, et al. Automated choroidal segmentation of 1060 nm OCT in healthy and pathologic eyes using a statistical model. Biomed Opt Express. 2012;3:86-103.
16. Mahajan NR, Donapati RC, Channappayya SS, et al. An automated algorithm for blood vessel count and area measurement in 2-D choroidal scan images. Conf Proc IEEE Eng Med Biol Soc. 2013;2013:3355-3358.
17. Kakileti S, Channappayya SS, Richhariya A, Chhablani J. An automated algorithm for the identification of choriocapillaris in 2D-OCT Images. Annual meeting of the SPIE; San Diego, CA; February 2014.